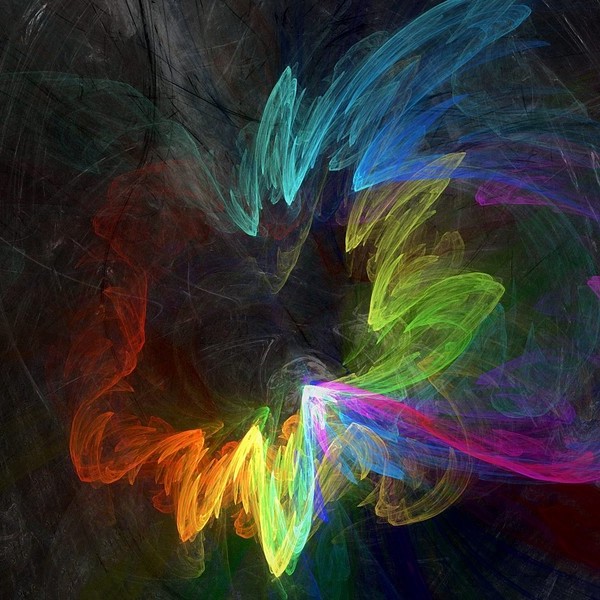
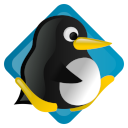
The .bin and .cue file are the parts of the actual game disc that you want. The .bin file contains almost all of the data and the .cue file contains some extra information about the structure of the CD. All the rest is Internet Archive stuff (and an image of the game cover of course).
To open it, you can convert it to a .iso disk image instead, which any Linux distribution can open as if it were a real CD. This blog post talks about how to do that. The last paragraph about mount
you can probably replace with double-clicking the .iso file in the GUI I would guess.
This, and see also “minmaxing,” the process of optimizing something (usually your character in a game) to get minimum penalty and/or maximum benefit, usually ignoring anything like realism or storytelling and focusing entirely on the stats and numbers.